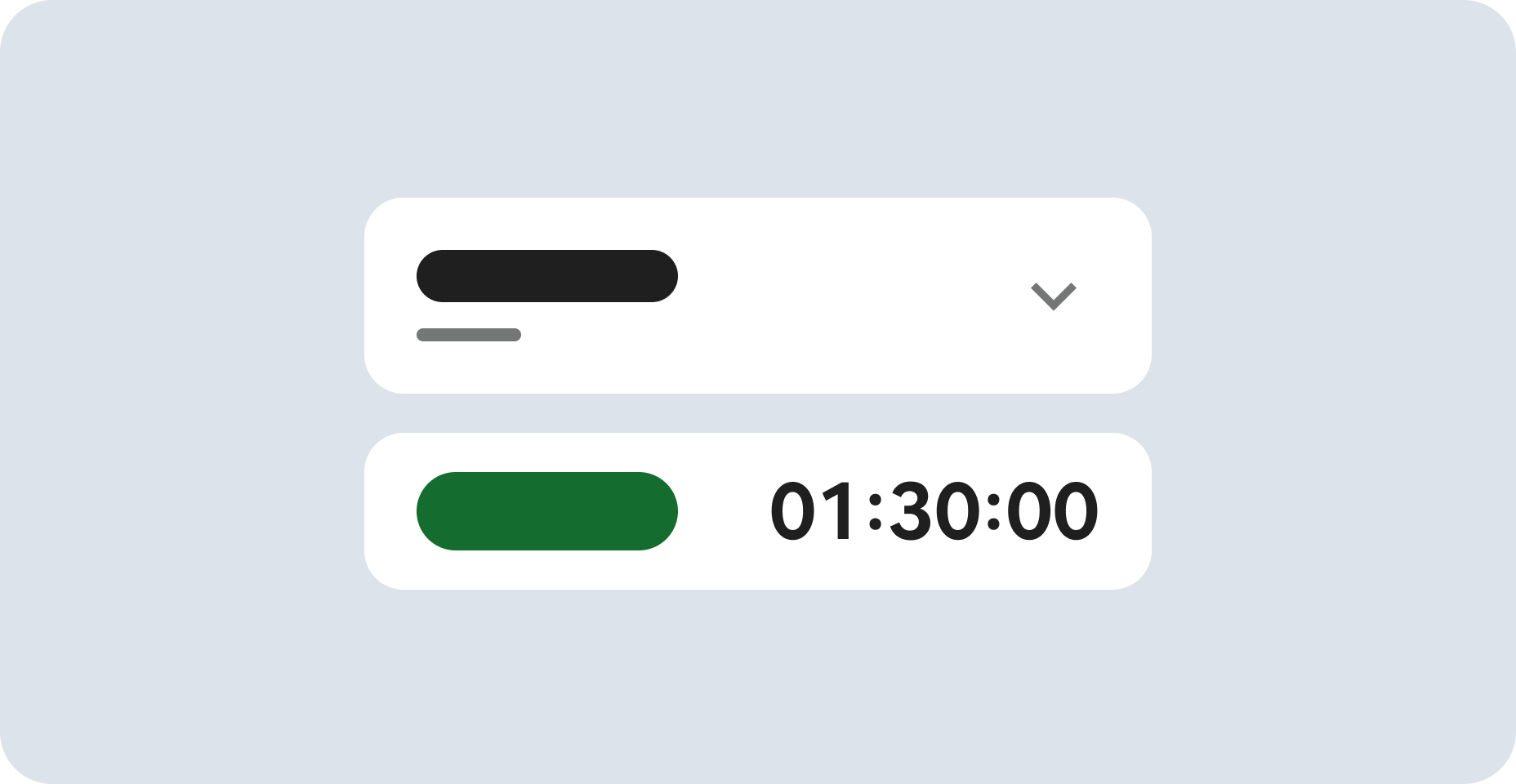
Before you begin
- Labs create a Google Cloud project and resources for a fixed time
- Labs have a time limit and no pause feature. If you end the lab, you'll have to restart from the beginning.
- On the top left of your screen, click Start lab to begin
Install Vertex AI SDK for Python and import libraries
/ 10
Generate a simple weather function call
/ 30
Generate a complex function call
/ 30
Generate function calls from a chat prompt
/ 30
Function calling lets developers create a description of a function in their code, then pass that description to a language model in a request. The response from the model includes the name of a function that matches the description and the arguments to call it with.
Function calling is similar to Vertex AI Extensions in that they both generate information about functions. The difference between them is that function calling returns JSON data with the name of a function and the arguments to use in your code, whereas Vertex AI Extensions returns the function and calls it for you.
Gemini is a family of powerful generative AI models developed by Google DeepMind, capable of understanding and generating various forms of content, including text, code, images, audio, and video.
The Gemini API in Vertex AI provides a unified interface for interacting with Gemini models. This allows developers to easily integrate these powerful AI capabilities into their applications. For the most up-to-date details and specific features of the latest versions, please refer to the official Gemini documentation.
Before starting this lab, you should be familiar with:
In this lab, you learn how to:
gemini-1.5-pro
) model:
Read these instructions. Labs are timed and you cannot pause them. The timer, which starts when you click Start Lab, shows how long Google Cloud resources are made available to you.
This hands-on lab lets you do the lab activities in a real cloud environment, not in a simulation or demo environment. It does so by giving you new, temporary credentials you use to sign in and access Google Cloud for the duration of the lab.
To complete this lab, you need:
Click the Start Lab button. If you need to pay for the lab, a dialog opens for you to select your payment method. On the left is the Lab Details pane with the following:
Click Open Google Cloud console (or right-click and select Open Link in Incognito Window if you are running the Chrome browser).
The lab spins up resources, and then opens another tab that shows the Sign in page.
Tip: Arrange the tabs in separate windows, side-by-side.
If necessary, copy the Username below and paste it into the Sign in dialog.
You can also find the Username in the Lab Details pane.
Click Next.
Copy the Password below and paste it into the Welcome dialog.
You can also find the Password in the Lab Details pane.
Click Next.
Click through the subsequent pages:
After a few moments, the Google Cloud console opens in this tab.
In the Google Cloud console, on the Navigation menu (), click Vertex AI > Workbench.
Find the
The JupyterLab interface for your Workbench instance opens in a new browser tab.
Open the
In the Select Kernel dialog, choose Python 3 from the list of available kernels.
Run through the Getting Started and the Import libraries sections of the notebook.
In the following sections, you will run through the notebook cells to see how to use the Gemini API in Vertex AI with the Vertex AI SDK for Python.
Click Check my progress to verify the objective.
When working with a generative text model, it can be difficult to coerce the LLM to give consistent responses in a structured format such as JSON. Function calling makes it easy to work with LLMs via prompts and unstructured inputs, and have the LLM return a structured response that can be used to call an external function.
You can think of function calling as a way to get structured output from user prompts and function definitions, use that structured output to make an API request to an external system, then return the function response to the LLM to generate a response to the user. In other words, function calling in Gemini extracts structured parameters from unstructured text or messages from users. In this example, you'll use function calling along with the chat modality in the Gemini model to help customers get information about products in the Google Store.
Click Check my progress to verify the objective.
In this example, you'll use the text modality in the Gemini API to define a function that takes multiple parameters as inputs. You'll use the function call response to then make a live API call to convert an address to latitude and longitude coordinates.
Click Check my progress to verify the objective.
In the previous examples, you made use of the entity extraction functionality within Gemini Function Calling so that you could pass the resulting parameters to a REST API or client library. However, you might want to only perform the entity extraction step with Gemini Function Calling and stop there without actually calling an API. You can think of this functionality as a convenient way to transform unstructured text data into structured fields.
In this example, you'll build a log extractor that takes raw log data and transforms it into structured data with details about error messages.
Click Check my progress to verify the objective.
Congratulations! In this lab, you learned how to use the Gemini API in Vertex AI to generate function calls from text prompts. You used the Gemini Pro model to generate function calls to help customers get information about products in the Google Store, geocode addresses, and extract entities from log data.
Check out the following resources to learn more about Gemini:
...helps you make the most of Google Cloud technologies. Our classes include technical skills and best practices to help you get up to speed quickly and continue your learning journey. We offer fundamental to advanced level training, with on-demand, live, and virtual options to suit your busy schedule. Certifications help you validate and prove your skill and expertise in Google Cloud technologies.
Manual Last Updated October 8, 2024
Lab Last Tested October 8, 2024
Copyright 2025 Google LLC All rights reserved. Google and the Google logo are trademarks of Google LLC. All other company and product names may be trademarks of the respective companies with which they are associated.
This content is not currently available
We will notify you via email when it becomes available
Great!
We will contact you via email if it becomes available
One lab at a time
Confirm to end all existing labs and start this one